Diese Domain wurde erfolgreich für einen Kunden von
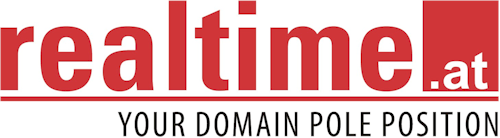
gecatcht!
realtime.at ist NICHT Inhaber dieser Domain, diese Domain kann über uns nicht mehr erworben werden.
Für Fragen zur Domain wenden Sie sich bitte direkt an den Domaininhaber laut Whois.
|
Wir unterstützen derzeit folgende Endungen:
.AT (Österreich)
.BE (Belgien)
.CH (Schweiz)
.DE (Deutschland)
.EU (Europäische Union)
.LI (Liechtenstein) |
|